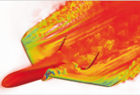
Proceedings
home
preface
contents
authors
keywords
copyright
reference
©2012 Civil-Comp Ltd |
 |
 |
 |
T. Mareš, E. Janouchová and A. Kucerová
Department of Mechanics, Faculty of Civil Engineering, Czech Technical University in Prague, Czech Republic
Keywords: artificial neural network, multi-layer perceptron, approximation, parameter identification, affinity hydration model, cement paste.
full paper (pdf) -
reference
A variety of engineering tasks leads to an inverse analysis
problem. Generally, the aim of an inverse analysis is to rediscover
unknown inputs from the known outputs. In common engineering
applications, a goal is to determine the initial conditions and
properties from physical experiments or, equivalently, to find a set
of parameters for a numerical model describing properly the
experiment.
While the numerical model of an experiment represents a well-defined
mapping from input (model, material, structural, or other) parameters
to output (structural response), there is no guarantee that the
inverse relation even exist. In engineering practice the inverse
relation is often ill-posed, highly nonlinear and multi-modal. Therefore,
the choice of an appropriate identification strategy is not trivial.
Moreover, such identification process is supposed to be performed
repeatedly for any new measurement and therefore, the emphasis is also
placed on the efficiency of the chosen identification method.
Overall, there are two main philosophies to the solution of
identification problems [1]. A forward
(classical) mode-direction is based on the definition of an error
function of the difference between outputs of the model and
experimental measurements. A solution comes with the minimisation of this
error function. In the case of complex time-consuming models, the
optimisation process can become easily unfeasible. Such a situation can be
solved by introducing some approximation (surrogate or meta-) model.
The second philosophy, an inverse mode, assumes the existence of an
inverse relationship between outputs and inputs. If such relationship
is established, then the retrieval of the desired inputs is a matter of
seconds and could be easily executed repeatedly. On the contrary, the
main disadvantage is an exhausting search for the inverse
relationship. Generally, this inverse model does not need to exist.
Nowadays, artificial neural networks (ANN) have became the most
frequently used methods for the approximation of nonlinear
relationships. In parameter identification problems they can be
efficiently applied in both described identification modes. In the
forward mode, ANN can be used to approximate the model reponse, while
in the inverse mode they can approximate the inverse relation between
model inputs and model outputs. In this paper, both
applications are presented for parameter identification of the affinity
hydration model for cement paste [2].
- 1
- A. Kucerová, "Identification of nonlinear mechanical model parameters based on soft computing methods", Ecole Normale Supérieure de Cachan, Laboratoire de Mécanique et Technologie, Ph.D. thesis, 2007.
- 2
- V. Šmilauer, "Multiscale Modeling of Hydrating Concrete", Saxe-Coburg Publications, UK, 2012. (To appear)
|