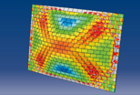
Proceedings
home
preface
contents
authors
keywords
copyright
reference
©2012 Civil-Comp Ltd |
 |
 |
 |
J.S. Angelo1, H.S. Bernardino1 and H.J.C. Barbosa1,2
1National Laboratory for Scientific Computing, LNCC / MCTI, Petropolis RJ, Brazil
2Federal University of Juiz de Fora, UFJF, MG, Brazil
Keywords: ant colony, multi-objective, structural optimization, constraint.
full paper (pdf) -
reference
Optimization techniques are fundamental tools in structural design, indicating the best ways in which a designer or a decision maker can extract the maximum from the available resources. As a result of the increasing competitiveness in industry and consequently, of the performance of its products, the design and analysis of novel optimization techniques in general, and nature inspired techniques in particular, are being widely explored.
Nature inspired metaheuristics can help overcome the challenges of the structural optimization problems, such as multiple objectives, discrete valued design variables, low regularity of the objective function(s), a large number of nonlinear implicit constraints, and expensive and, or unreliable gradients. In fact, besides the constraint in the stress of the bars, the problem of optimization of a framed structure can be formulated considering multiple and conflicting objective functions, such as the weight of the structure and the displacement of its nodes. Also, the design variables are often to be chosen from a list of commercially available sizes leading to a discrete search space. Thus, the use of nature inspired techniques becomes attractive.
Among the metaheuristics, the ant colony algorithms are probabilistic techniques inspired by the collective behaviour of ants and used for solving optimization problems. In the case of multi-objective problems the ant colony optimization (ACO) deals with the objectives separated from each other (using one pheromone matrix per objective), trying to find a set of efficient solutions, rather than a single one.
A common way to handle constraints when using nature inspired metaheuristics for optimization is to penalize the value of the objective(s) function(s) according to the level of constraint violation. However, it is not an easy task to combine these values to generate a unique performance measure. Here, a penalty scheme is used, which adaptively sizes each penalty coefficient using information collected during the search.
In this paper, two multi-objective ant colony approaches, namely the multi-objective ant system (MOAS) and the multi-objective ant colony system (MOACS), equipped with an adaptive penalty method that automatically adjusts the penalty coefficients are proposed to solve discrete and multi-objective structural optimization problems in which the weight of the structure and the displacements of its nodes are both to be minimized. The computational experiments are composed by five structural optimization problems, namely, the 10-, 25-, 60-, 72-, and 942-bar truss structures found in the literature. The results obtained are discussed and multi-objective performance metrics, including the empirical attainment function, are used to analyse the quality of the results generated.
|